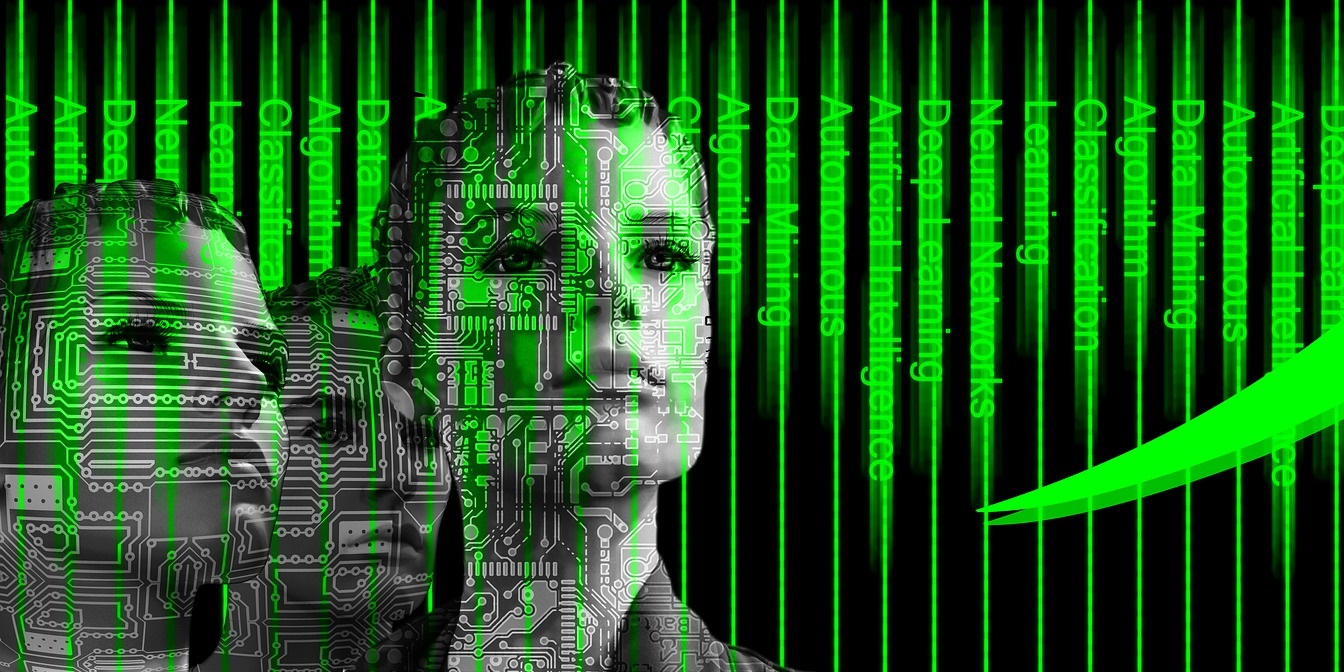
The interest in artificial intelligence (AI) is growing apace. Scarcely a day goes by without the publication of some article in the press concerning its abilities. However, many of these articles, particularly in the mainstream media, remain sensationalist and do not come to grips with the real potential for artificial intelligence in the business, and particularly, industrial sectors.
Aiming to provide more clarity for our readers, we interviewed Luca Formaggia, Professor of Numerical Analysis at the Politecnico di Milano, Italy, and President of SIMAI (the Italian Society of Industrial and Applied Mathematics) and Pierluigi Contucci, Professor of Mathematical Physics at the University of Bologna, and Coordinator of the National Research Plan (2019) on AI, Robotics and Cybersecurity.
![]() |
![]() |
Luca Formaggia | Pierluigi Contucci |
Q: In the widest engineering context, what are the real capabilities of AI as it stands today?
PC: The term AI today most often refers to the methods that have emerged in machine learning and deep learning in the last 10 years. In reality, AI consists of two main approaches, both of which began in the Fifties. There is classical AI, which consists of classical programs in different programming languages such as object oriented (OO) programs or those that use symbolic or sub symbolic methods. I can illustrate classical AI with an example from autonomous driving. A number of car manufacturers are particularly advanced in this area, for instance Elon Musk’s Tesla. As the car drives with an inactive driver inside it, it is constantly observing the road ahead. If it suddenly “sees” an unexpected obstacle such as a stationery car ahead of it in the road, the vehicle must apply its brakes. This decision to brake is made by classical AI which follows “if… then…” reasoning – if I am proceeding at a certain speed and I draw closer and closer to the stationery object, then I will apply the brakes.
The other main approach in AI today, called machine learning or deep learning, also started in the Fifties, but has experienced a boom more recently due to the increase in computer processing power and the availability of large databases. To go back to the example of autonomous driving, machine learning here is being used to “see” where pedestrians are, to recognize a pedestrian that is crossing the road, to identify that it is a human, and so on. These methods are known as machine learning because no classical AI program exists that can say whether the obstacle in the road is a human or an inanimate object.
These activities are done by machine learning which has a whole series of capabilities that are being developed along three principal lines: the first is image recognition; the second is language recognition, specifically spoken language, written language and text translation, etc.; and a third category, which is a growing ability to profile buying customers – the great promise of marketing. This third area, which is experiencing very rapid growth, is already being used by the largest organizations such as Amazon and Google and is far less publicized because it obviously directly transforms into revenue for these companies, giving them a competitive advantage. There are thousands of examples of these three main categories, some of which are very spectacular.
LF: At the moment neural networks work well at classification. If I, as a human being, need to identify whether the obstacle in front of my car is a pedestrian, or distinguish a cat or a dog in a photo, the cerebral processes (which, incidentally, is where the name neural networks originates) used are largely unknown and extremely complex.
Deep neural networks are trying to replicate these processes in a simplified way. If there is sufficient, good quality data available, then they can be trained to achieve results as efficiently, or even more efficiently, than the human brain. While this is extraordinary from a technical perspective, it is not really surprising. I will explain with an analogy: if I use a calculator to perform an operation on two numbers of ten digits each, the calculator gives me the result in a millisecond. This means my simple calculator is much superior to the human brain in this area. So, by extrapolation, it really should not surprise us that other algorithms like deep learning methods may be able to achieve specific results better than humans can.
I believe the problem for these technologies arises from some of the more superficial articles about AI that almost imbue them with the superior intelligence of understanding – as if the self-driving car “understands” what it is doing. Let me be quite clear: there is NO understanding. There is simply an algorithm that is highly competent in executing certain functions. Perhaps machine understanding may be possible in some far distant future, but for now, fortunately, understanding is something that is uniquely human. Understanding lies with the creator of the machine and with the person who can correctly interpret the results that the machine generates, and who can then instruct the machine, because the machine needs to be taught.
Every now and again one sees sensationalist articles that make outrageous claims for AI. The most recent that I saw claimed that a neural network had “discovered” Kepler’s laws of planetary motion! This was not true. The neural network had simply correctly fitted certain parameters together based on certain information that it had been previously given. Kepler instead created his theory based on very little data, just a few observations, after which he followed a process of logical reasoning and deduction to formulate the laws governing the motion of the planets – he did not just simply fit together certain data.
This brings me back to a topic that was discussed in the workshop at the 35th International CAE Conference in October 2019, and that is this: these methods are not a substitute for knowledge; they are not a substitute for the engineer who understands how to perform a good simulation, or the fluid dynamics of a hydraulic system.
On the contrary, I believe these machine learning AI techniques will be extremely useful, especially from an industrial perspective, if we integrate them with our prior knowledge, but not if we expect them to replace it.
So, in my opinion, one of the most important things for readers to understand is that existing knowledge can certainly leverage these techniques because they allow different types of analysis and are capable of analyzing huge quantities of data much more effectively and efficiently than a human could ever do. But they achieve their greatest purpose if they are integrated with prior knowledge and experience.
A neural network trained on a large quantity of data including and excluding defects would ultimately be able to identify a potential defect better than a human being could. But the ability to understand WHY the defect occurred and HOW to correct it requires an understanding that goes way beyond what these networks will be capable of doing for at least the next decades.
Q: A recent article by McKinsey Global Institute [1] states that Greenfield AI solutions are prevalent in business areas such as customer service management, and in healthcare, in particular. What is your take on this?
PC: I agree with McKinsey.
The medical field is a very promising area of direct applications. An example that has been circulating just recently is very topical. A neural network trained in China with the data from CAT scans of patients’ lungs has a high probability of recognizing the coronavirus in just minutes, as opposed to the time required for the entire procedure of taking a swab from the person. Since diagnostic imaging is an important part of medicine today, it is obvious that an automated tool that can recognize an image and classify it quickly falls directly within the realms of greenfield AI, as expressed by McKinsey.
Autonomous driving is very impressive, even though it is not imminent in the short term. This is not because of technological reasons, but for legal reasons in the sense that there are still no laws that govern autonomous driving on public roads. Autonomous driving today faces a similar phase to motor cars just after their introduction in the 18th Century: there was a long, slow period of adjustment of the laws. Insurance, for instance, was not compulsory in the beginning, but became obligatory over time because, occasionally, drivers would cause such serious accidents that they could not cover the damages. So, in a similar way, how would insurance have to be adapted to the issues of autonomous driving? This gigantic lag in legal adaptation means that the impetus of the pace of technological development will eventually force the launch of autonomous driving, overcoming the delays arising from the institutional difficulties in instituting the rules to govern it.
LF: The prerequisites for any AI application to work successfully are the availability of sufficient, significant data and expert guidance. It is insufficient to have a lot of data – it must be significant data. If placing 100 sensors, as opposed to 30 or 40, simply generates more of exactly the same information, then that extra amount of data is not helpful and makes absolutely no difference. While this is a trivial observation, it is sometimes overlooked: you need the right type of data, with informational content in a technical sense, to train a neural network effectively.
Medicine represents an enormous field of possibility – image processing is a substantial area where neural networks have already been used successfully. AI is already assisting diagnostic imaging in the automatic recognition of tumors and other pathologies. But we should also not ignore the neural network’s ability to integrate or somehow interpret seemingly minor data. For instance, the new health monitors in smart watches can give us our heart rate, metabolic rate, and other simple data, such as body temperature. Training a neural network with such data may help doctors detect possible pathological situations using “hidden” information that was not obvious or recognized beforehand. This is possible and could definitely provide some enormous advantages to medical diagnosis or monitoring.
There are also some other fields of application that will have an impact over a longer time. They are currently more niche and the subject of active research and involve the integration of previous knowledge with deep learning. Simulation algorithms based on physical principles are particularly good at calculating, for instance, the displacement of a bridge under a certain load or the efficiency of a wing. So how can we take advantage now of these new techniques? In many ways. For example, we can train a neural network for the better characterization of materials, especially new, complex composite materials, where it is difficult to find constitutive relationships simply.
Machine learning can also help with various difficult numerical aspects. Some of my colleagues work with neural networks applied to turbulence models where the calculations are very complex and require enormous computing power. If AI can be applied to that, it can improve our capabilities. For instance, AI can provide the best parameters to put into a computational fluid dynamic code for a given flow condition. This may allow one to improve the predictive capabilities of the numerical simulations and enable their use in fields where they were not employed before because they were too expensive or inaccurate.
Q: Is there enough trust in the mathematical models used by AI? I am thinking for example of the Boeing airplanes that had to be grounded because of the repeated number of accidents, in spite of the numerical analyses of the performance of the planes and their components.
PC: It is important to distinguish between human trust and institutional trust. They are two different things that will proceed along independent tracks. The legal tools to “certify” a machine learning program will have to be developed over time, while machines will earn the trust of human beings in time and, if they make some mistakes, they will “pay” for them with a loss of trust. It is important to acknowledge and understand that machine learning is not a science yet. I always draw a parallel with the industrial revolution and thermodynamics. At the beginning of the industrial revolution, steam engines were invented and worked. But no one knew why they worked because thermodynamics was not yet understood. Physicists and mathematicians generated the theory of thermodynamics to explain why steam engines worked. It provided a series of laws, a few principles, from which the mechanical object’s operation and efficiency could be deduced. This is what is now required for machine learning. We need a theory that clarifies why it works and determine its efficiency. This is the work that is currently being undertaken by mathematicians and physicists and to which Luca alluded when he said we want to transform this set of empirical knowledge which is very interesting and even functional, but which cannot yet be described with rigorous tools. A lot of these competences still need to be developed, and there has to be a joint venture between governments and industry to promote it.
I would like to appeal to business decision makers to finance research into this topic. Business has much to gain from these instruments, and so should contribute to their development and to the development of skills and education in this area. To develop the field, we will need new courses in artificial intelligence, and we will need to train people to use and program it.
LF: We definitely need to better understand deep learning so that it can be used with sufficient trust in critical areas where one needs more precise bounds on the results. Until then, we will still need human decision makers to make the final decisions.
Q: What are some of the important areas of future development around machine learning?
PC: In addition to those we have already mentioned, one huge area is the interpretability of the results that are generated. There is a great effort to integrate machine learning’s predictive ability with interpretation and explanation skills. Answering the “Why?” questions: Why did you give me that answer? Let us suppose that from the data emitted by this machine one could predict, just a few seconds before it does so, that it will break and at that point there. While this predictive information is valuable, the machine owner would want to move beyond that to the why: why were you able to predict it? An experienced technician may hear a change in the noise the machine makes and would stop it. Upon investigation, he may find a crack. But machine learning sometimes does a lot more and we often do not know why the neural network gives the correct predictions and response that it does. This enormous effort into interpretability and explainability is an unexplored frontier of machine learning.
I have no illusions; however, I think there are pockets of machine learning that will always remain strangely difficult to explain. In explaining this to my students, I draw a parallel with a human – even a child – that sees a cat and can immediately identify it as a cat instead of a dog. But, why is that identified as a cat and this other animal as a dog? A human will say, “Ah, because a dog is bigger. A cat moves one way and it meows, while a dog barks,” – incidentally, the barking is a sound input – but there is no classical computer program to which one can explain how to recognize a cat as a cat and a dog as a dog. A neural network’s ability to recognize these two animals and to distinguish between them is one of the most impressive things about machine learning. So, explainability in the recognition of an image meets intrinsic obstacles. I do not think everything can be explained to learning machines. I think a lot can be translated into explanations and interpreted in the way we mean, but there will be a whole range of correct answers from machine learning that have no explanation because they are far removed from classical coding. The coding would say: If a parameter is satisfied, then I can make a conclusion.
But what is the “if” parameter that tells me it is a cat in the picture. We just do not know.
LF: Neural networks want to imitate the functioning of the human brain, albeit in a hyper-simplified manner. But we humans also do many things without understanding in the least why we can do them. For instance, we learn how to walk naturally, but we know very little about how our brains learn that. This fact also applies to the interpretability and explainability of the results generated by neural networks. We observe that in several situations they work extremely well, but we still have to understand why. It is extraordinarily complex, and I agree that perhaps we will never be able to explain it fully.
These are issues that are less visible in newspaper articles. The scientific community is trying to understand how and why neural networks work. Understanding will help us to design better algorithms or decide which type of AI tool should be used for a specific problem, and what its properties and limitations are. The design of a neural network today is still often done by trial and error. In a sense, we are still at the Wright brothers’ stage – trying a longer wing or a different shape until the plane takes off. We still have to get to the stage of aviation today where we know the Navier Stokes equations and have a clear idea of why planes fly. There is a very big gap to close around the “why” in machine and deep learning, which is what is keeping many mathematicians and physicists busy in this field.
Q: Some people tend to think of AI as only being available and valuable for large organizations. What is your opinion on this?
PC: The Italian economy is characterized by SMEs that are largely represented by Confindustria. Typically, these small industrial realities have several tens or a few hundred to a few thousand employees. For instance, around Bologna, the entire mechanical sector and all its satellite businesses consists of mid-sized companies. AI can do fantastic things for these companies.
To give you a concrete example, we did some work with the company GD Italy. It is not really a large mechanical company, but they are the world leaders in their area. They make machines for high-speed cigarette making and packing, and related solutions for the tobacco industry. Tons of tobacco arrive at the assembly line and the tobacco has to leave the factory already rolled into cigarettes which have been packed into individual boxes of cigarettes, that in turn have been packed into cartons of cigarettes, which have been packed into bulk packaging. For the cigarette companies, it is vital that this assembly line is smooth and continuous. GD builds enormous high-speed packing machines that take care of this entire packaging assembly line. For them, the ability to predict or foresee a malfunction or a breakdown is something that has an incalculable value. Until now, this has been done by technicians that supervise the machines as they work. Now, however, with the enormous quantity of data available, a diagnostic method for error or failure prediction can be developed. The quality of the data is essential, however. More sensors located in relevant points in the machine can provide the necessary data, and it is the human technician who is best able to suggest where to place these sensors. So, integrating human competence with machine learning capacity is essential.
Q: There are many disparate individual national attempts and some coordinated international attempts being made to create a policy approach for AI. At what point are we in Italy with regard to a policy governing the regulation and use of AI?
PC: The academic and scientific communities are very aware of the potential of AI and of what needs to be done. For structural reasons, everywhere in the world, institutions are always late. Now, imagine how late they could be in a country where, on average, a government lasts one year at a time, and each new government almost always effectively denounces and annuls everything done by the preceding government. Regulating an important policy approach in this type of political environment, which has gone from bad to worse since the Second World War, is incredibly difficult. However, there is a huge sense of urgency around problem solving among individuals in this country, so there is no need to be pessimistic. There is a strong awareness that this topic needs to be governed, and while this work will be protracted and will face many obstacles, we should be able accomplish something successfully thanks to focused work being done by small groups in the country.
References
[1] McKinsey Global Institute - Notes from the AI frontier: Applications and value of deep learning https://www.mckinsey.com/featured-insights/ artificial-intelligence/notes-from-the-ai-frontier-applications-and-value of-deep-learning